Natural Language-based Financial Forecasting
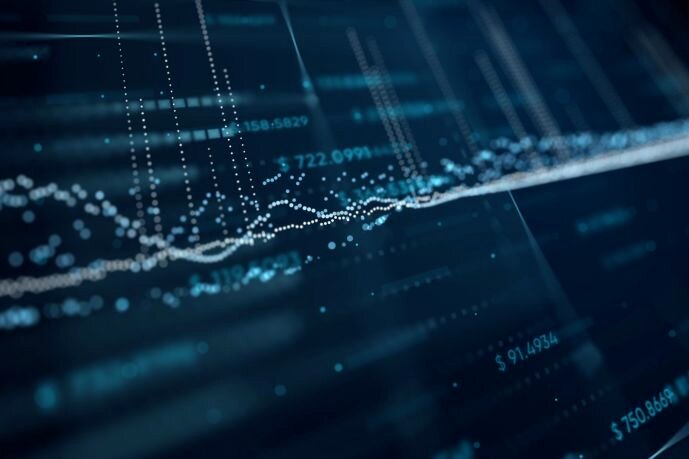
Natural language-based financial forecasting (NLFF) is an emerging field that lies at the intersection of natural language processing (NLP) and financial forecasting. NLFF encompasses several areas, including semantic computing, natural language understanding, and time series analysis. The term NLFF was first introduced by Nassirtoussi et al. (2014) and is fueled by the growing volume of financial reports, press releases, news articles, and social media.
Figure 1 demonstrates the exponential growth of scientific papers focused on NLFF in Scopus between 1998 and 2016. In Indonesia, there has been a recent uptick in NLFF-related scientific publications.
- Cakra and Trisedya (2015) applied feature extraction of sentiment and linear regression for the 13 registered stocks on Twitter, to model the Jakarta Composite Index (JCI) for the period of 14th to 30th April 2015 and
- Saputro (2019) conducted research on predicting the JCI and Rupiah exchange rate against the dollar on Twitter, utilizing a combination of sentiment analysis and the average of TF-IDF calculation for the period of 27th May – 27th July 2018. The data modeling in Saputro’s research involved the use of the least absolute shrinkage and selection operator (LASSO) and model averaging
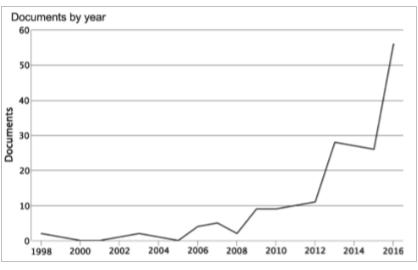
Sentiment analysis is a crucial component of natural language-based financial forecasting (NLFF) as it helps extract and obtain relevant information from textual data and apply it to financial data analysis. This can be challenging due to the complexity of financial language and the need for accurate interpretation. To address this challenge, various approaches have been employed, such as knowledge-based techniques, statistical methods, and hybrid approaches. Each of these methods has its own advantages and limitations, and choosing the appropriate approach depends on the specific needs and goals of the analysis.
For further details, I invite you to visit my Github repository!
Fortunately, I have extensively researched this topic during my last year of study in the Department of Statistics at IPB University. I invite you to explore my findings
Sources